HSBI researcher develops AI for more efficient cell segmentation - good for pharmaceutical research, for example
Images of segmented cells are extremely important in biomedical research and diagnostics. And enormously expensive. Although artificial intelligence (AI) can now also perform cell segmentation, it requires training with a large amount of labeled data: Data that has to be manually labeled by experts. PhD student Eiram Mahera Sheikh at Bielefeld University of Applied Sciences is researching how this process can be intelligently accelerated as part of the SAIL research network. She is already using AI, or more precisely, deep active learning, to prepare the training data.
The two images called up in the computer are images of the same biological cells. On the left, the microscopic image of some pancreatic stem cells on a porous substrate: the small white spots, of different sizes, elongated in shape, slightly blurred against a gray background, are the individual cells. It is not so easy to tell whether a pixel still belongs to the cell or is already part of the background. Eiram Mahera Sheikh draws visitors' attention to the right-hand side: the same arrangement of cells, but this time differently colored and clearly delineated from the now black background. "With this mask, the image is much easier to use for analysis. Here, the cells are clearly segmented with the help of AI, or artificial intelligence." Sheikh wants to ensure that this process can be done even more efficiently in the future - by making the AI smart using AI. In other words, she wants to enable the necessary training of the cell segmentation AI to take place more quickly than before, using an AI process.
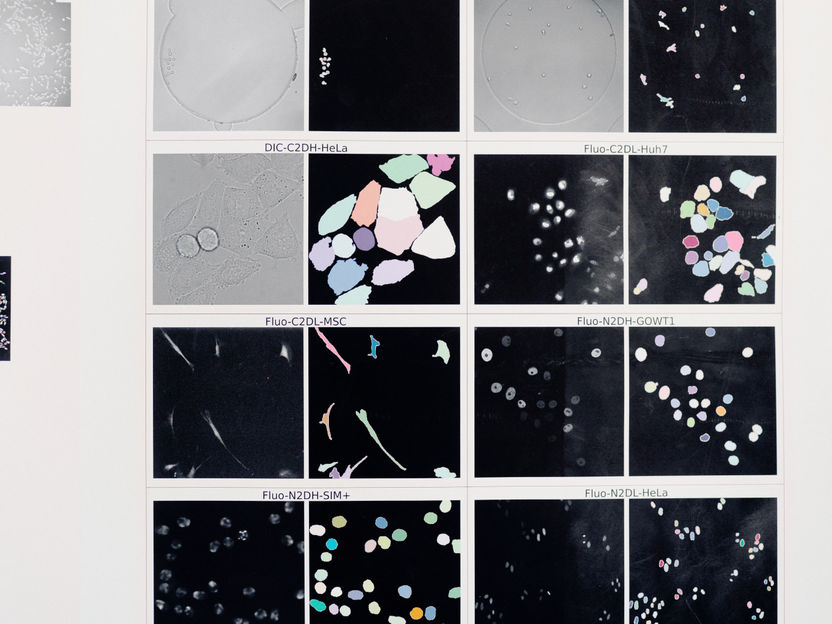
The AI should identify image regions that are particularly important for learning cell segmentation. This would mean that all image pixels would no longer need to be labeled by hand, but only those that are particularly valuable as training data.
P. Pollmeier/HSBI
Valuable for cancer therapy, among other things: cell segmentation provides insights into changes at cell level
But first things first: After several years in the profession, the 33-year-old computer scientist with Indian roots specialized in data science and AI as part of a master's degree. She has now joined Bielefeld University of Applied Sciences (HSBI) as a doctoral student. With cell segmentation, she has chosen a topic that is of great importance in biomedical research and diagnostics: "Analyzing images of segmented cells provides important insights into the characteristics of cells, pathological changes or how cells react to certain active substances," explains Eiram Mahera Sheikh. "This is information that is important in cancer therapy or pharmacological research, for example." However, Sheikh's research starts two steps earlier - on a meta-level, so to speak. "Cell segmentation can now also be performed by AIs. I'm interested in training this AI." And that's quite something...
PhD on cell segmentation is being carried out as part of the OWL research network SAIL
Prof. Dr. Wolfram Schenck is sitting in a meeting room at HSBI. He listens intently to Sheikh's presentation and looks at the images projected onto the wall. The doctoral student's interim results are being discussed. The project is part of the interdisciplinary research network SAIL. This stands for SustAInable Life-Cicle of Intelligent Socio-Technical Systems - roughly translated: Sustainable Life-Cycle of Intelligent Socio-Technical Systems. In the network, which is funded by the state of North Rhine-Westphalia with up to 14.8 million euros, Bielefeld University as consortium leader, HSBI, TH OWL and Paderborn University are cooperating with the aim of making AI systems transparent, safe, sustainable and robust over their entire life cycle. To this end, a 360-degree view of AI is being taken: The network's 90 or so scientists are looking at basic research in the field of AI as well as its impact on people and society and specific applications in the field of Industry 4.0 and smart healthcare. Wolfram Schenck, Professor of Engineering Informatics, Scientific Director of the Data Analytics Cluster of the Center for Applied Data Science (CfADS) at the Gütersloh campus and HSBI spokesperson for the SAIL project, is supervising Eiram Mahera Sheik's doctorate, which is located at the interface of basic research and healthcare, but also provides insights beyond this.
Labeling of image data: How can time be saved by highly specialized experts?
Schenck explains the challenges of Sheik's work: "In order to learn cell segmentation, the cell segmentation AI needs a lot of labeled data for training. This is image data at pixel level that is provided with additional information and classified." A complex process, because in concrete terms this means that for each pixel of an image of biological cells, it must be determined whether it belongs to the "background" class, the "membrane" class or the "cell body" class, for example. Schenck explains: "Today, this labeling can only be done manually by experts who know exactly what is to be seen." Cell membrane? Dead cell? Background? - Pixel by pixel, someone who knows something about the subject has to do the tedious, somewhat monotonous work before the AI can be made fit for the segmentation of certain cells.
"Because the work is so time-consuming and monotonous, the experts are reluctant to do it," interjects Dr. Constanze Schwan. "Apart from that, labeling is also very expensive due to the lengthy process and the use of highly trained specialists." Schwan joined the discussion. The computer scientist on HSBI's Career@BI program not only teaches in the Department of Engineering and Mathematics, but also works in the research department of the biotech company Miltenyi Biotec in Göttingen. She brings practical needs to the table: "It would be a great step forward if there were a model or algorithm that would reduce the effort required for labeling." This is because existing segmentation methods, which are suitable for autonomous driving for example, reach their limits in the case of microscopic images. This is partly due to the more complex and finer structures found in the images. These are often image stacks on a time axis for 3D structures. "These images or image stacks often contain complex structures that are difficult to segment," explains Dr. Alaa Othman, who completes the team. As a junior research group leader in the SAIL network, the electrical engineer is supervising Sheikh's doctorate together with Prof. Schenck.
Progress in sight: Sheikh's AI sorts the unimportant training data from
In order to get around the problem of complex structures and to relieve the specialist teams that have to train the cell segmentation AI, Eiram Mahera Sheikh relies on AI. "My aim is to identify the image regions that are particularly important for learning cell segmentation. This would mean that all image pixels would no longer have to be labeled by hand, but only those that are particularly valuable as training data." Such targeted picking of potential training data is called "active learning", explains Schenck: "And because the AI algorithms for cell segmentation come from deep learning, we also refer to this as 'deep active learning'."
Sheikh has now identified the most valuable training data: "These are the most uncertain ones, the ones where the AI algorithm has difficulties, for example due to poor image contrast or cells that overlap or have an irregular shape." Only these should therefore be looked at and labeled by experts in the future, while the effort could be saved in the clear cases because Sheik's AI has already done the labeling. "Basically, the cell segmentation AI learns with less data and yet so effectively that it can still make precise cell segmentations later," summarizes Alaa Othman.
Transfer of the findings to other applications possible, e.g. to autonomous driving
The question remains as to how the importance of the potential training data is determined. Should the entire image be considered? Or are local and sectional decisions needed? The group delves into the discussion, which a layperson is soon no longer able to follow. "Ultimately, the project is application-oriented basic research," says Wolfram Schenck. This is because the algorithms for deep active learning developed by Eiram Mahera Sheikh, which divide the image data into important and unimportant regions for AI training and thus make cell segmentation more effective and efficient overall, can also be used for other applications, for example in medical imaging, autonomous driving or in the analysis of satellite images. Schenck: "Once again, the holistic and sustainable approach of the SAIL project becomes clear."
Note: This article has been translated using a computer system without human intervention. LUMITOS offers these automatic translations to present a wider range of current news. Since this article has been translated with automatic translation, it is possible that it contains errors in vocabulary, syntax or grammar. The original article in German can be found here.
Other news from the department science
Most read news
More news from our other portals
See the theme worlds for related content
Topic world Diagnostics
Diagnostics is at the heart of modern medicine and forms a crucial interface between research and patient care in the biotech and pharmaceutical industries. It not only enables early detection and monitoring of disease, but also plays a central role in individualized medicine by enabling targeted therapies based on an individual's genetic and molecular signature.
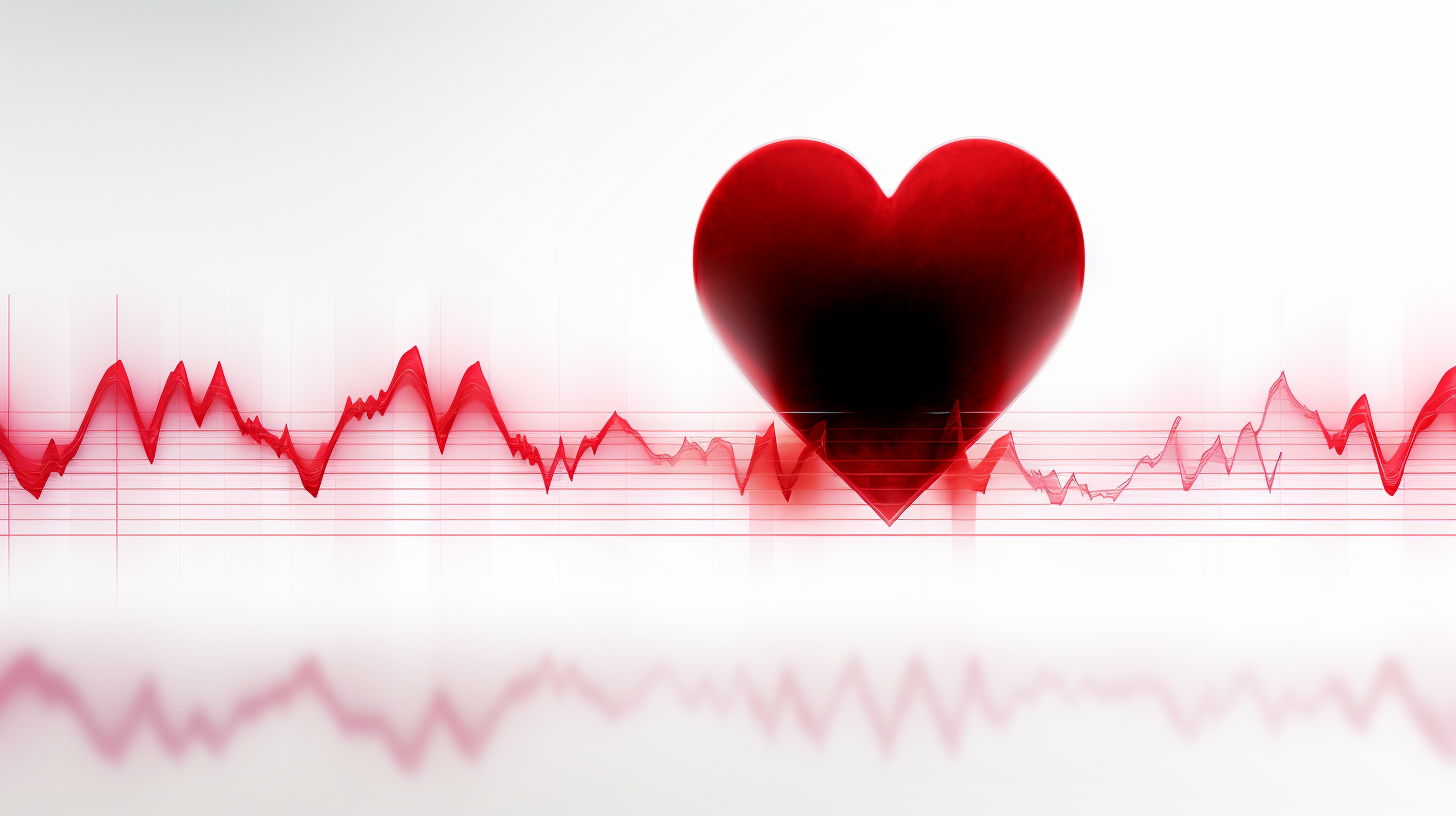
Topic world Diagnostics
Diagnostics is at the heart of modern medicine and forms a crucial interface between research and patient care in the biotech and pharmaceutical industries. It not only enables early detection and monitoring of disease, but also plays a central role in individualized medicine by enabling targeted therapies based on an individual's genetic and molecular signature.