How thoughts influence what the eyes see
A surprising study could point to new approaches for AI systems
When you see a bag of carrots at the grocery store, does your mind go to potatoes and parsnips or buffalo wings and celery?
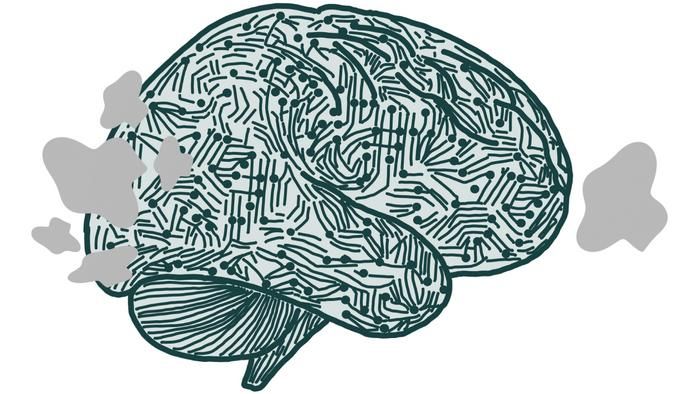
Early visual areas in the brain adapt their representations of the same visual stimulus depending on what task we're trying to perform.
Rungratsameetaweemana lab/Columbia Engineering
It depends, of course, on whether you’re making a hearty winter stew or getting ready to watch the Super Bowl.
Most scientists agree that categorizing an object — like thinking of a carrot as either a root vegetable or a party snack — is the job of the prefrontal cortex, the brain region responsible for reasoning and other high-level functions that make us smart and social. In that account, the eyes and visual regions of the brain are kind of like a security camera collecting data and processing it in a standardized way before passing it off for analysis.
However, a new study led by biomedical engineer and neuroscientist Nuttida Rungratsameetaweemana, an assistant professor at Columbia Engineering, shows that the brain’s visual regions play an active role in making sense of information. Crucially, the way it interprets the information depends on what the rest of the brain is working on.
If it’s Super Bowl Sunday, the visual system sees those carrots on a veggie tray before the prefrontal cortex knows they exist.
Published in Nature Communications, the study provides some of the clearest evidence yet that early sensory systems play a role in decision-making — and that they adapt in real-time. It also points to new approaches for designing AI systems that can adapt to new or unexpected situations.
Rungratsameetaweemana explains more about the research:
What’s exciting about this new study?
Our findings challenge the traditional view that early sensory areas in the brain are simply “looking” or “recording” visual input. In fact, the human brain’s visual system actively reshapes how it represents the exact same object depending on what you’re trying to do. Even in visual areas that are very close to raw information that enters the eyes, the brain has the flexibility to tune its interpretation and responses based on the current task. It gives us a new way to think about flexibility in the brain and opens up ideas for how to potentially build more adaptive AI systems modeled after these neural strategies.
How did you come to this surprising conclusion?
Most previous work looked at how people learn categories over time, but this study zooms in on the flexibility piece: How does the brain rapidly switch between different ways of organizing the same visual information?
What were your experiments like?
We used functional magnetic resonance imaging (fMRI) to observe people’s brain activity while they put shapes in different categories. The twist was that the “rules” for categorizing the shapes kept changing. This let us determine whether the visual cortex was changing how it represented the shapes depending on how we had defined the categories.
We analyzed the data using computational machine learning tools, including multivariate classifiers. These tools allow us to examine patterns of brain activation in response to different shape images, and measure how clearly the brain distinguishes shapes in different categories. We saw that the brain responds differently depending on what categories our participants were sorting the shapes into.
What did you see in the data from these experiments?
Activity in the visual system — including the primary and secondary visual cortices, which deal with data straight from the eyes — changed with practically every task. They reorganized their activity depending on which decision rules people were using, which was shown by the brain activation patterns becoming more distinctive when a shape was near the grey area between categories. Those were the most difficult shapes to tell apart, so it’s exactly when extra processing would be most helpful.
We could actually see clearer neural patterns in the fMRI data in cases when people did a better job on the tasks. That suggests the visual cortex may directly help us solve flexible categorization tasks.
What are the implications of these findings?
Flexible cognition is a hallmark of human cognition, and even state-of-the-art AI systems currently still struggle with flexible task performance. Our results may contribute to designing AI systems that can better adapt to new situations. The results may also contribute to understanding how cognitive flexibility might break down in conditions like ADHD or other cognitive disorders. It’s also a reminder of how remarkable and efficient our brains are, even at the earliest stages of processing.
What’s next for this line of research?
We’re pushing the neuroscience further by studying how flexible coding works at the level of neural circuits. With fMRI, we were looking at large populations of neurons. In a new follow-up study, we are investigating the circuit mechanisms of flexible coding by recording neurological activity inside the skull. This lets us ask how individual neurons and neuronal circuits in the human brain support flexible, goal-directed behavior.
We’re also starting to explore how these ideas might be useful for artificial systems. Humans are really good at adapting to new goals, even when the rules change, but current AI systems often struggle with that kind of flexibility. We’re hoping that what we’re learning from the human brain can help us design models that adapt more fluidly, not just to new inputs, but to new contexts.