New AI tool for brain tumor diagnostics
A deep-learning algorithm that can automatically detect and evaluate brain tumors on PET scans
A team at Forschungszentrum Jülich has developed a deep-learning algorithm that can automatically detect and evaluate brain tumors on PET scans. In a fraction of the time, the AI achieves results comparable to those of an experienced physician, the team reports in the Journal of Nuclear Medicine.
At initial diagnosis and during treatment, it is important to determine the extent and volume of a brain tumor as accurately as possible. Can the tumor be operated on? Will it respond to treatment or continue to grow? The method of choice initially is magnetic resonance imaging (MRI) because it accurately detects structural changes. However, structural abnormalities do not necessarily reflect the true extent of the tumor. In many cases, a variant of positron emission tomography (PET) provides results that differ from MRI, as studies have shown. However, evaluating PET scans in terms of tumor extent takes a lot of time, which is why this is rarely done routinely in the clinic. The team led by study leader Philipp Lohmann from the Institute of Neuroscience and Medicine (INM-4) at Forschungszentrum Jülich has now developed a new deep-learning algorithm that automatically detects tumors in the PET data and determines their volume. This makes the analysis much faster while maintaining the quality of the results. "The AI tool we developed could help physicians obtain important diagnostic information that was rarely available before," Lohmann says.
PET uses radioactively labeled biomolecules to make certain metabolic processes visible. Radioactively labeled amino acids have proven particularly useful for imaging brain tumors. The rapidly growing cancer cells absorb the amino acids much faster than healthy brain cells. "On PET images, the enriched amino acids often allow us to determine a different or larger tumor extent than with MRI," explains Lohmann. In this context, experts speak of so-called metabolic tumor volume, because the measurement method is based on metabolic processes of the cells. During evaluation, however, a physician must determine the tumor outlines on several dozen slice images of a PET scan. "That takes a lot of time, which is why the method is rarely performed in clinical practice, despite its high informative value," Lohmann knows.
To change that, researchers from Juelich, Heidelberg and Cologne developed a deep-learning algorithm called "JuST BrainPET" (Juelich Segmentation Tool for Brain Tumor PET) that evaluates amino acid PET images fully automatically in terms of metabolic tumor volume. The algorithm was trained using 476 PET datasets and subsequently tested on 223, all of which had arisen during initial diagnosis or during follow-up examinations of a total of 555 brain tumor patients. The associated tumor volume information came from analyses performed by experienced nuclear medicine physicians.
"Our AI has learned to distinguish the tumor from other structures that also take up more of the amino acids for physiological reasons - such as vessels or muscle tissue," says Robin Gutsche, a doctoral student at INM-4 and first author of the study, who was instrumental in developing the AI algorithm. "It works even when the tumors are directly adjacent to these structures." Within minutes, the algorithm detects the tumor and determines the metabolic tumor volume. The results agree very well with the values determined by the experts.
The researchers additionally tested the clinical utility of the algorithm by having it assess the treatment success of chemotherapy in patients with gliomas. "Here, the goal was to answer questions such as: How well does a patient respond to therapy, or what is his prognosis?" said Lohmann. "We were able to show that the AI does the clinical assessment just as well as a specialist - but in a fraction of the time." However, AI should not replace the physician, but support him, he emphasizes.
Furthermore, the new AI approach could be used to better standardize the evaluation of PET measurements to make results more comparable between different clinics and institutions. "We hope that our algorithm will encourage treating physicians in neuro-oncology centers to use amino acid PET more frequently in their patients - even if they have little experience with the method," Lohmann says. JuST_BrainPET is available for free on the Github platform.
Note: This article has been translated using a computer system without human intervention. LUMITOS offers these automatic translations to present a wider range of current news. Since this article has been translated with automatic translation, it is possible that it contains errors in vocabulary, syntax or grammar. The original article in German can be found here.
Original publication
Other news from the department science
Most read news
More news from our other portals
See the theme worlds for related content
Topic world Diagnostics
Diagnostics is at the heart of modern medicine and forms a crucial interface between research and patient care in the biotech and pharmaceutical industries. It not only enables early detection and monitoring of disease, but also plays a central role in individualized medicine by enabling targeted therapies based on an individual's genetic and molecular signature.
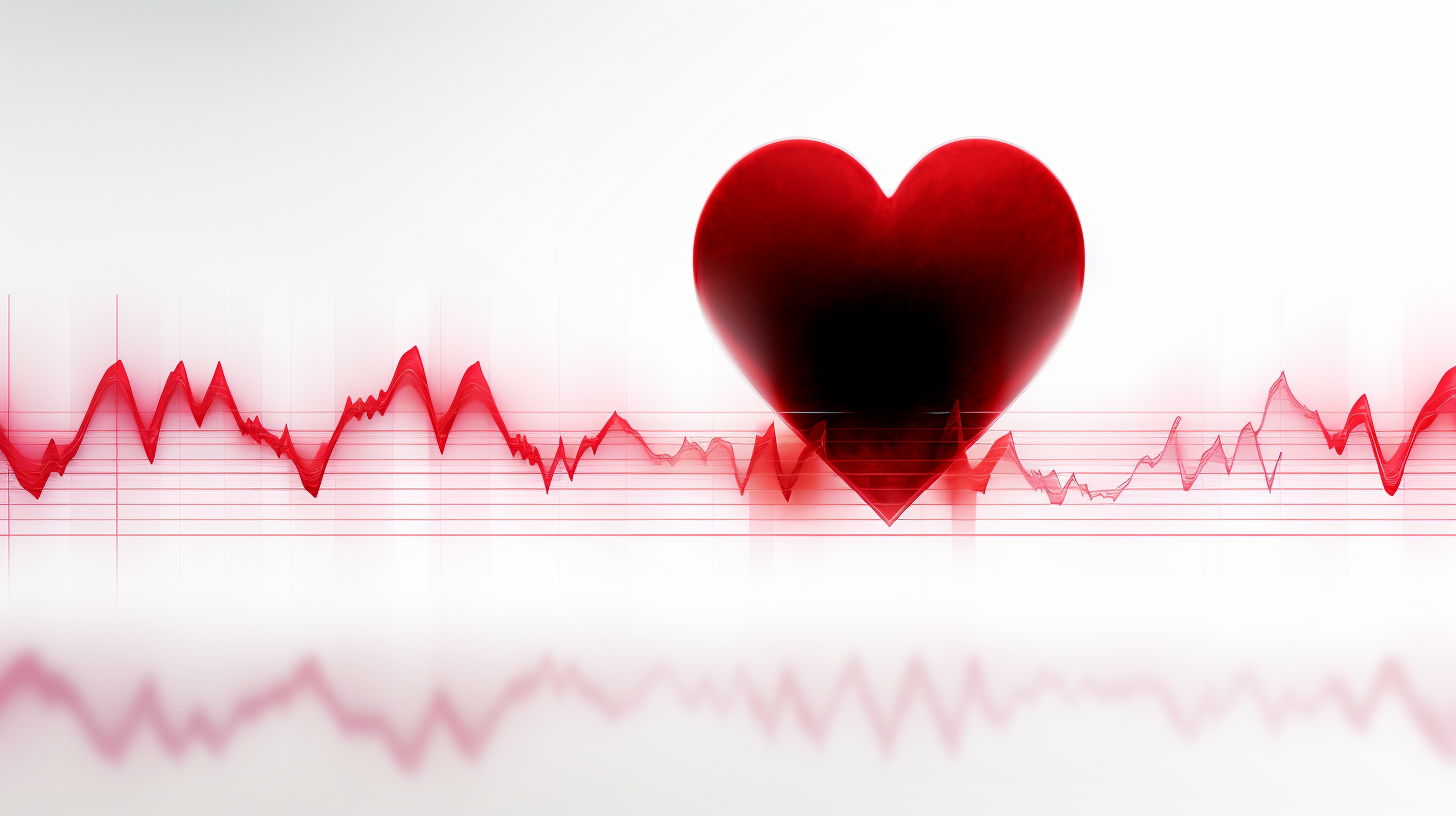
Topic world Diagnostics
Diagnostics is at the heart of modern medicine and forms a crucial interface between research and patient care in the biotech and pharmaceutical industries. It not only enables early detection and monitoring of disease, but also plays a central role in individualized medicine by enabling targeted therapies based on an individual's genetic and molecular signature.