Trust is good, but AI control is better
Hybrid graph AI optimizes material use along the value chain and assessment tools check AI systems for potential weaknesses
What are the worst-case scenarios if certain materials are not available? What are suitable substitutes? Can we optimize the use of materials with as little waste as possible? And how do we achieve our sustainability goals? "This is where our material graph comes in. Our AI learns not only from texts or images about material, properties or intended use, but also from knowledge and practical know-how to identify material risks for production or services, to manage supply chains or parts requirements and to optimize material use and purchasing", explains Hendrik Stange, Head of the team Auto Intelligence at Fraunhofer IAIS in Sankt Augustin. For example, the AI keeps track of a possible expiration date of a material so that it is used before the due date. Or it permanently forecasts required quantities to save ways or to produce materials cost-efficiently.
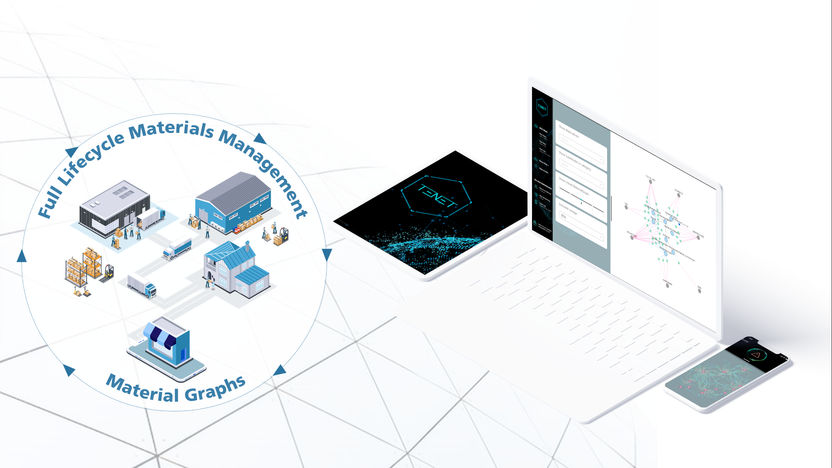
A material graph is like a road network in materials management, through which artificial intelligence guides you like a kind of navigation system. The goal is optimized and sustainable material consumption as well as cost reduction.
Fraunhofer IAIS / SBN Data Technologies
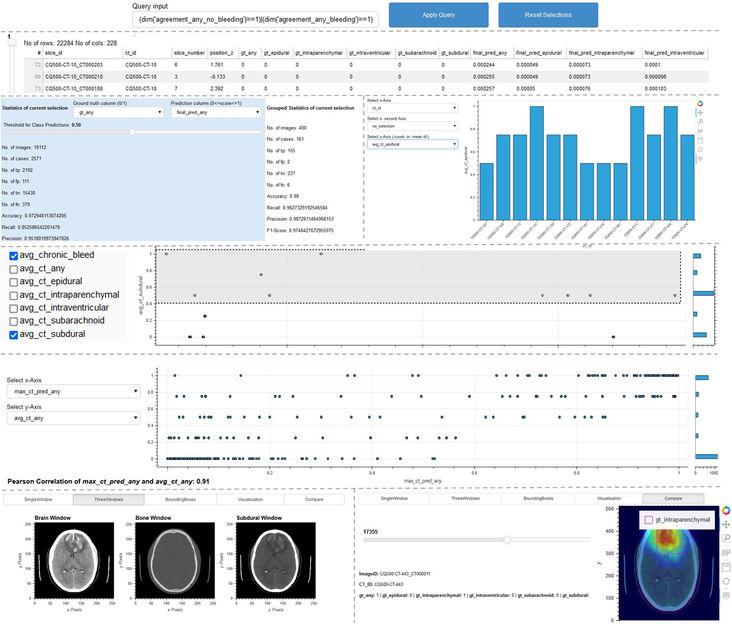
The ScrutinAI tool is used to detect errors in AI models or training data and to analyze the causes. In this example, an AI model for detecting abnormalities and diseases in CT images is being studied.
Fraunhofer IAIS
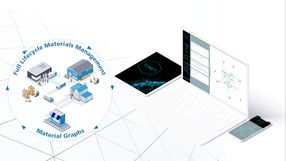
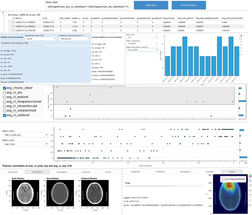
But how exactly does a material graph work? "Think of it as a road network of your material management, through which artificial intelligence guides us like a kind of navigation system to reach your destinations. If the experts want the most ecological route from start to finish, the AI recommends a route that can save carbon dioxide, for example by saving trips to deliver or use the material. If the experts are looking for an immediate effect, the graph AI will suggest the best way to achieve this effect in the form of an optimized mix of materials", explains Stange.
At Hannover Messe, visitors to the joint Fraunhofer booth can experience a material graph by the example of a wound care provider. "Our solution approach puts industrial AI on a completely new level. From the upstream supply chain to the application, the entire use of materials can be optimized to improve cost-effectiveness and, in the best case, shorten wound healing. At the same time, the material quantities that are expected to be needed during a therapy become transparent to keep surplus and transport costs as low as possible", explains the expert.
Material graphs can be adapted to different industries: Hybrid AI combines machine intelligence with human intelligence from experts to train AI applications even with small data. The main goal is to avoid waste, optimize material consumption, reduce costs and anticipate supply bottlenecks. Hendrik Stange: "Our AI is a sparring partner for professionals to help them make informed decisions more quickly, supported by profound recommendations. It's not the AI that decides whether to take route A or B. That remains in the power of humans."
Assessment tools for a safe, secure and trustworthy use of AI
Dr. Maximilian Poretschkin is represented at the joint Fraunhofer booth with a different focus. The Head of safe AI and AI certification at Fraunhofer IAIS will be showcasing various assessment tools and methods that examine and evaluate artificial intelligences in terms of their reliability, fairness, robustness and transparency. The test criteria are based on the AI Assessment Catalog, a guideline for the design of trustworthy artificial intelligence, which was published by the Fraunhofer IAIS experts in 2021. Since January 2023 it is also available in an English translation.
But why is it so important to be able to systematically test the quality and validity of AI applications? "Especially in sensitive fields of application, such as medical diagnostics, HR management, finance, the applications used by law enforcement agencies or safety-critical areas, AI systems must deliver absolutely reliable results. The AI Act – the European draft for regulating AI systems – ranks many of these examples in the high-risk category and even requires mandatory assessment in these cases", explains Poretschkin. "Companies that develop or use high-risk AI applications urgently need to address how they can ensure the quality of their applications."
The challenge is that AI works differently than conventional software. The latter is programmed on the basis of rules, thereby enabling systematic testing of its functionality – i.e. whether the responses or outputs are correct in relation to the inputs. For AI applications, these procedures are in general not sufficient, especially if they are based on neural networks.
The "ScrutinAI" tool developed by Fraunhofer IAIS enables test personnel and assessors to systematically search for vulnerabilities in neural networks and thus assess the quality of AI applications. One specific example is an AI application that detects abnormalities and diseases in CT images. The question here is whether all types of abnormalities are detected equally well, or whether some are detected better than others. This analysis helps test personnel assess whether an AI application is suitable for its intended context of use. At the same time, developers can also benefit by being able to identify insufficiencies in their AI systems at an early stage and take appropriate improvement measures, such as enhancing the training data with specific examples.
Modular software framework offers support for the selection and assessing of AI systems
Further assessment tools and procedures are integrated into a software framework and can be combined with each other in a modular way. For example, the so-called "benchmarking" tool can be used to examine which AI model is best suited for a specific task. "There is a glut of new AI applications that companies can integrate into their processes. Benchmarking helps them to make the right choice," says the researcher. The "uncertAInty" method can also be used to assess how confident a neural network is about the result it produces. It may be that a neural network is very uncertain because it has received input data that it does not yet know from previous training data. "For example, an autonomous vehicle must be able to reliably detect objects and people in its environment so that it can react to them appropriately. The uncertainty assessment helps in measuring how much you can trust the system´s decision, whether certain fallback mechanisms need to be activated or if a human needs to make the final decision," explains Poretschkin.
From April 17 to 21, visitors of Hannover Messe 2023 are able to experience various assessment tools and processes live from experts of the Fraunhofer IAIS at the joint Fraunhofer booth A12 in Hall 16. Hendrik Stange is Head of the team Auto Intelligence, which focuses on automated AI in material management: This AI builds on innovative material graphs to make companies resource aware and empower them to be more economically forward-thinking and ecologically sustainable. Dr. Maximilian Poretschkin, Head of safe AI and AI certification, uses various assessment tools to show how to evaluate the quality of AI applications and explains why this is becoming increasingly important.