Artificial intelligence folds RNA molecules
New structures reliably predictable
The spatial structure of RNA molecules is crucial for their function. But predicting it is a challenge.
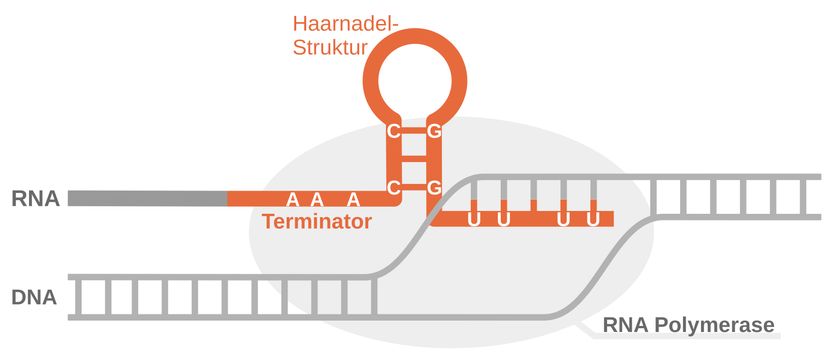
Some segments of an RNA molecule (orange) form so-called hairpin structures.
© Vivian Brandenburg
For the function of many biomolecules, their three-dimensional structure is crucial. Researchers are therefore not only interested in the sequence of the individual building blocks of biomolecules, but also in their spatial structure. With the help of artificial intelligence (AI), bioinformaticians can already reliably predict the three-dimensional structure of a protein from its amino acid sequence. For RNA molecules, however, this technology is still in its infancy. Researchers at Ruhr-Universität Bochum (RUB) describe a way to use AI to reliably predict the structure of certain RNA molecules from their nucleotide sequence in the journal PLOS Computational Biology of 7 July 2022.
For the work, the teams led by Vivian Brandenburg and Professor Franz Narberhaus from the RUB Chair of Biology of Microorganisms cooperated with Professor Axel Mosig from the Bioinformatics Competence Area of the Bochum Centre for Protein Diagnostics.
Cell environment must be taken into account
“RNA is often only seen as a messenger between genomic DNA and proteins”, says Axel Mosig. “But many RNA molecules take over cellular functions.” Their spatial structure is important for this. Similar regions in a nucleotide sequence can cluster together to form three-dimensional arrangements.
“Identifying these self-similarities in an RNA sequence is like a mathematical puzzle”, explains Vivian Brandenburg. There is a biophysical model for this puzzle with corresponding prediction algorithms. However, the model cannot take into account the cellular environment of the RNA – and this also influences the folding process. “If the RNA were isolated and floating in aqueous solution, the model could predict the structure very accurately”, says Brandenburg. But a living cell contains many other components.
This is where artificial intelligence comes into play. The AI can learn subtle patterns from the cellular environment based on known structures. It could then incorporate these findings into its structural predictions. For the learning process, however, the AI needs sufficient training data – and this is actually lacking in practice.
Obtaining training data with a trick
To solve the problem of the missing training data, the Bochum team used a trick: the researchers worked with known RNA structural motifs. Using a kind of reverse gear, they could generate almost any number of nucleotide sequences from the energy models of these structures that would fold into these spatial structures. With the help of this so-called inverse folding, the researchers generated many pairs of nucleotide sequences and structures with which they could train the AI.
New structures reliably predictable
The researchers then confronted the AI with a new task: it had to predict the structure of certain bacterial RNA molecules. These molecules – called transcription terminators – are important stop signals in the translation of genomic DNA in bacteria. Often, like many other RNA molecules with important cellular functions, they are hidden in the genome and difficult to distinguish from areas with other functions.
The artificial intelligence was able to reliably recognise and predict the typical structure of the transcription terminators, which is reminiscent of a hairpin. The research team was able to prove this using publicly available experimental data.
“While AI approaches are now almost inevitable in the prediction of protein structures, the development of RNA structures is only just beginning”, Axel Mosig summarises.