Machine learning radically reduces workload of cell counting for disease diagnosis
The use of machine learning to perform blood cell counts for diagnosis of disease instead of expensive and often less accurate cell analyzer machines has nevertheless been very labor-intensive as it takes an enormous amount of manual annotation work by humans in the training of the machine learning model. However, researchers at Benihang university have developed a new training method that automates much of this activity.
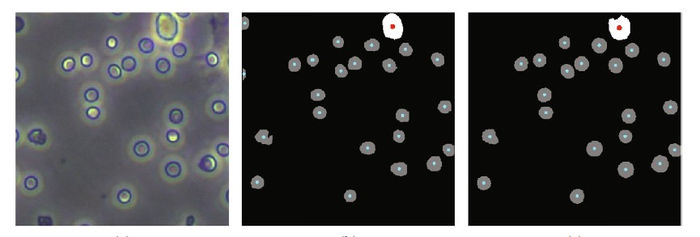
Results of cell counts compared to predicted count results showed that the newly developed training method allows machine learning to more accurately count blood cells
Cyborg and Bionic Systems
Their new training scheme is described in a paper published in the journal of Cyborg and Bionic Systems on April 9.
The number and type of cells in the blood often play a crucial role in disease diagnosis, but the cell analysis techniques commonly used to perform such counting of blood cells—involving the detection and measurement of physical and chemical characteristics of cells suspended in fluid—are expensive and require complex preparations. Worse still, the accuracy of cell analyzer machines is only about 90 percent due to various influences such as temperature, pH, voltage, and magnetic field that can confuse the equipment.
In order to improve accuracy, reduce complexity and lower costs, much research into alternatives has lately focussed on the use of computer programs to perform “segmentation” on photographs of the blood taken by a high-definition camera connected to a microscope. Segmentation involves algorithms that perform pixel-by-pixel labeling of what appears in a photo, in this case, what parts of the image are cells and which are not—in essence, counting the number of cells in an image.
For images in which only a single type of cell appears, such methods achieve a decent level of accuracy, but they perform poorly when confronting images with multiple types of cells. So in recent years, in attempts to solve the problem, researchers have turned to convolutional neural networks (CNNs)—a type of machine learning that mirrors the connection structure of the human visual cortex.
For the CNN to perform this task, it must first be “trained” to understand what is and is not a cell on many thousands of images of cells that humans have manually labeled. Then, when fed a novel, unlabelled image, it recognizes and can count the cells in it.
“But such manual labeling is laborious and expensive, even when done with the assistance of experts,” said Guangdong Zhan, a co-author of the paper and professor with the Department of Mechanical Engineering and Automation at Beihang University, “which defeats the purpose of an alternative that is supposed to be simpler and cheaper than cell analyzers.”
So the researchers at Beihang University developed a new scheme for training the CNN, in this case, U-Net, a fully convolutional network segmentation model that has been widely used in medical image segmentation since it was first developed in 2015.
In the new training scheme, the CNN is first trained on a set of many thousands of images with only one type of cell (taken from the blood of mice).
These single-cell-type images are “preprocessed” automatically by conventional algorithms that reduce noise in the images, enhance their quality, and detect the contours of objects in the image. They then perform adaptive image segmentation. This latter algorithm calculates the various levels of gray in a black and white image, and if a part of the image lies beyond a certain threshold of gray, the algorithm segments that out as a distinct object. What makes the process adaptive is that rather than segmenting out parts of the image segments according to a fixed gray threshold, it does this according to the local features of the image.
After the single-cell-type training set is presented to the U-Net model, the model is fine-tuned using a small set of manually annotated images of multiple cell types. In comparison, a certain amount of manual annotation remains, and the number of images needed to be labeled by humans drops from what was previously many thousands to just 600.
To test their training scheme, the researchers first used a traditional cell analyzer on the same mouse blood samples to do an independent cell count against which they could compare their new approach. They found that the accuracy of their training scheme on segmentation of multiple-cell-type images was 94.85 percent, which is the same level achieved by training with manually annotated multiple-cell-type images.
The technique can also be applied to more advanced models to consider more complex segmentation problems.
As the new training technique still involves some level of manual annotation, the researchers hope to go on to develop a fully automatic algorithm for annotating and training models.
Original publication
Other news from the department science
Most read news
More news from our other portals
See the theme worlds for related content
Topic World Cell Analysis
Cell analyse advanced method allows us to explore and understand cells in their many facets. From single cell analysis to flow cytometry and imaging technology, cell analysis provides us with valuable insights into the structure, function and interaction of cells. Whether in medicine, biological research or pharmacology, cell analysis is revolutionizing our understanding of disease, development and treatment options.
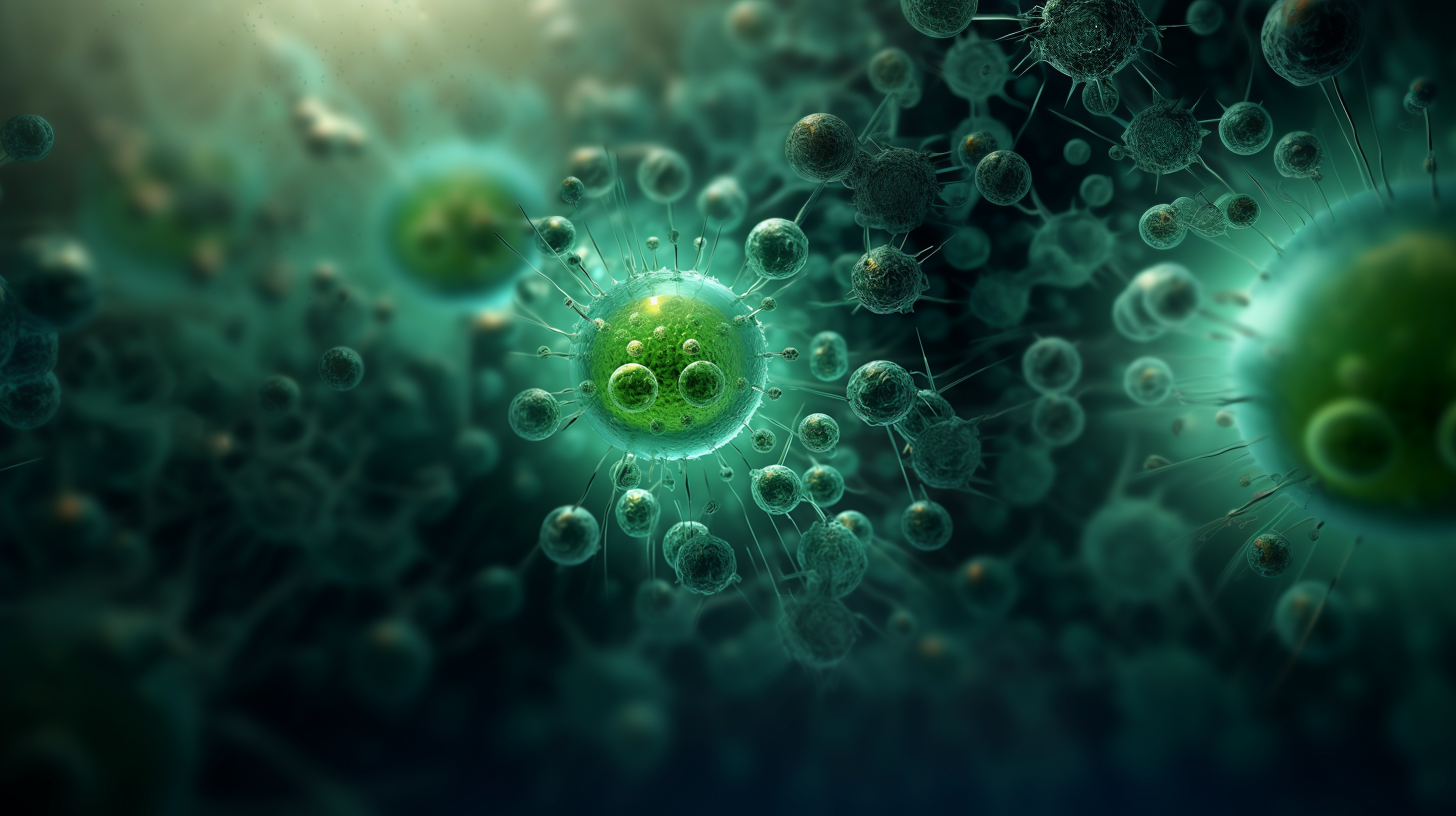
Topic World Cell Analysis
Cell analyse advanced method allows us to explore and understand cells in their many facets. From single cell analysis to flow cytometry and imaging technology, cell analysis provides us with valuable insights into the structure, function and interaction of cells. Whether in medicine, biological research or pharmacology, cell analysis is revolutionizing our understanding of disease, development and treatment options.