Deep learning dreams up new protein structures
Researchers show that a neural network trained exclusively to predict protein shapes can also generate new ones
Just as convincing images of cats can be created using artificial intelligence, new proteins can now be made using similar tools. In a report in Nature, a team including researchers at the University of Washington,Rensselaer Polytechnic Institute, and Harvard University describe the development of a neural network that “hallucinates” proteins with new, stable structures.
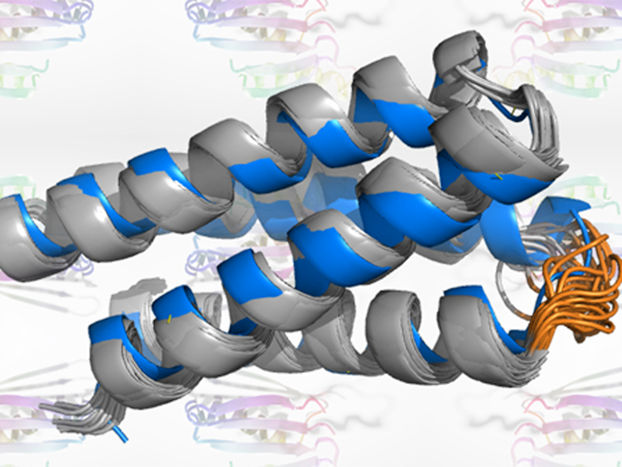
A neural network "hallucinated" proteins that were synthesized to confirm their structure.
Rensselaer Polytechnic Institute
“The potential to hallucinate brand-new proteins that bind particular biomolecules or form desired enzymatic active sites is very exciting,” said Gaetano Montelione, a professor of chemistry and chemical biology at Rensselaer, where synthesized versions of “hallucinated” proteins invented by a neural network were analyzed.
Proteins are string-like molecules found in every cell that spontaneously fold into intricate three-dimensional shapes. These folded shapes are key to nearly every process in biology, including cellular development, DNA repair, and metabolism. But the complexity of protein shapes makes them difficult to study. Biochemists often use computers to predict how protein strings, or sequences, might fold. In recent years, artificial intelligence techniques like neural networks and deep learning have revolutionized the accuracy of this work.
“For this project, we made up completely random protein sequences and introduced mutations into them until our neural network predicted that they would fold into stable structures,” said co-lead author Ivan Anishchenko, a postdoctoral scholar in the Baker lab in the Institute for Protein Designat the University of Washington School of Medicine. “At no point did we guide the software toward a particular outcome — these new proteins are just what a computer dreams up.”
In the future, the team believes it should be possible to steer the artificial intelligence so that it generates new proteins with useful features. “We’d like to use deep learning to design proteins with function, including protein-based drugs, enzymes, you name it,” said co-lead author Sam Pellock, a postdoctoral scholar in the Baker lab.
The research team generated 2,000 new protein sequences that were predicted to fold. Over 100 of these were produced in the laboratory and studied. Detailed analysis on three such proteins confirmed that the shapes predicted by the computer were indeed realized in the lab.
“Our solution NMR studies, along with X-ray crystal structures determined by the University of Washington team, demonstrate the remarkable accuracy of protein designs created by the hallucination approach,” said co-author Theresa Ramelot, a senior research scientist in the Montelione lab within the Rensselaer Center for Biotechnology and Interdisciplinary Studies.
Montelione notes, “The hallucination approach builds on earlier observations we made together with the Baker lab revealing that protein structure prediction with deep learning can be quite accurate even for a single protein sequence, without recourse to contact predictions usually obtained by analysis of many evolutionary-related protein sequences.”
“This approach greatly simplifies protein design,” said senior author David Baker, recipient of the 2021 Breakthrough Prize in Life Sciences. “Before, to create a new protein with a particular shape, people first carefully studied related structures in nature to come up with a set of rules that were then applied in the design process. New sets of rules were needed for each new type of fold. Here, by using a deep-learning network that already captures general principles of protein structure, we eliminate the need for fold-specific rules and open up the possibility of focusing on just the functional parts of a protein directly.”
“Exploring how to best use this strategy for specific applications is now an active area of research, and this is where I expect the next breakthroughs,” said Baker.