Deep learning enables early detection and classification of live bacteria using holography
Development of an AI-powered smart imaging system for early-detection and classification of live bacteria in water samples
Waterborne diseases affect more than 2 billion people worldwide, causing substantial economic burden. For example, the treatment of waterborne diseases costs more than $2 billion annually in the United States alone, with 90 million cases recorded per year. Among waterborne pathogen-related problems, one of the most common public health concerns is the presence of total coliform bacteria and Escherichia coli (E. coli) in drinking water, which indicates fecal contamination. Traditional culture-based bacteria detection methods often take 24-48 hours, followed by visual inspection and colony counting by an expert, according to the United States Environmental Protection Agency (EPA) guidelines. Alternatively, molecular detection methods based on, for example, the amplification of nucleic acids, can reduce the detection time to a few hours, but they generally lack the sensitivity for detecting bacteria at very low concentrations, and are not capable of differentiating between live and dead microorganisms. Furthermore, there is no EPA-approved nucleic acid-based method for detecting coliform bacteria in water samples.
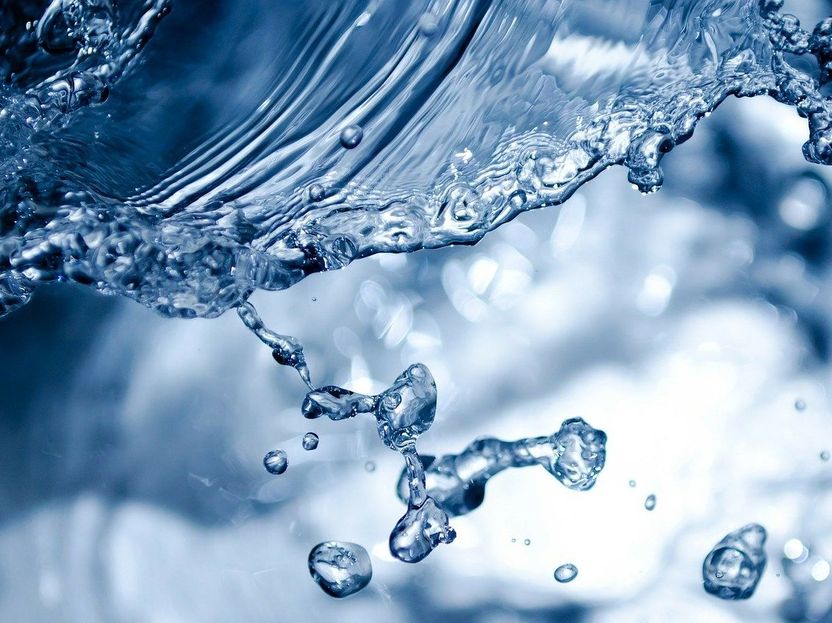
PublicDomainPictures/ Pixabay
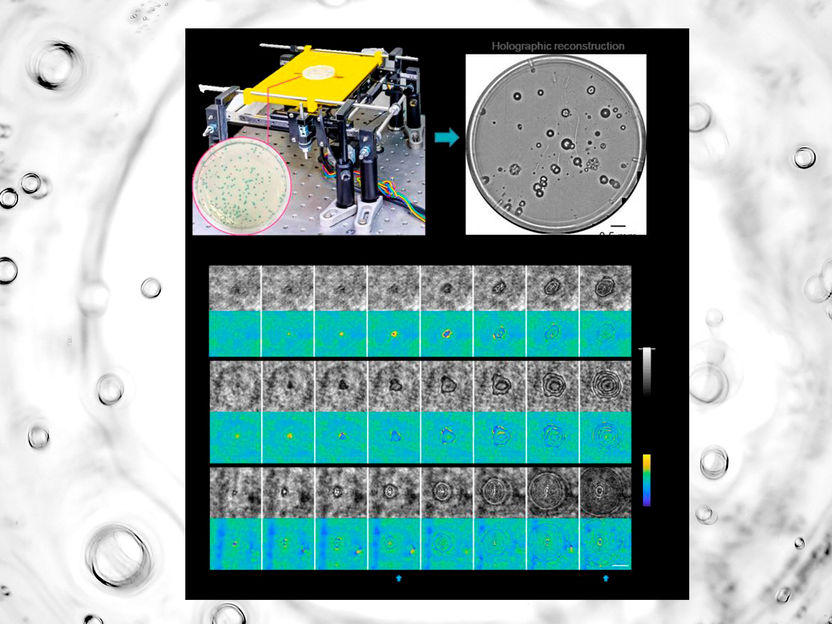
a, Schematic of the device. b, Whole plate image of E. coli and K. aerogenes colonies. c, Example images of the individual growing bacterial colonies detected by a trained deep neural network. The time points of the detection and classification of growing colonies are annotated with blue arrows. The scale bar is 0.1 mm. This research received funding from US ARO.
Hongda Wang, Hatice Ceylan Koydemir, Yunzhe Qiu, Bijie Bai, Yibo Zhang, Yiyin Jin, Sabiha Tok, Enis Cagatay Yilmaz, Esin Gumustekin, Yilin Luo, Yair Rivenson, Aydogan Ozcan
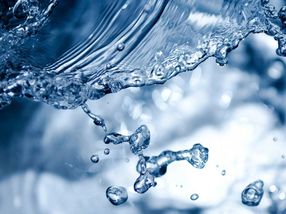
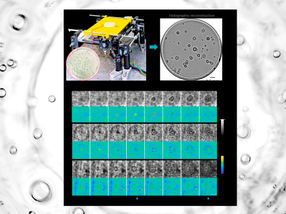
Therefore, there is an urgent need for an automated method that can achieve rapid and high-throughput bacterial colony detection with high sensitivity to provide a powerful alternative to the currently available EPA-approved gold-standard methods that take at least 24 hours and require an expert for colony counting.
In a new paper published in Light: Science & Applications, a team of scientists, led by Professor Aydogan Ozcan from the Electrical and Computer Engineering Department at the University of California, Los Angeles (UCLA), USA, and co-workers have developed an AI-powered smart imaging system for early-detection and classification of live bacteria in water samples. Based on holography, they designed a highly sensitive and high-throughput imaging system, which continuously captures microscopic images of a whole culture plate, where bacteria grow, to rapidly detect colony growth by analyzing these time-lapse images with a deep neural network. Following the detection of each colony growth, a second neural network is used to classify the type of bacteria.
The efficacy of this unique platform was demonstrated by performing early detection and classification of three types of bacteria, i.e., E. coli, Klebsiella aerogenes (K. aerogenes), and Klebsiella pneumoniae (K. pneumoniae), and the UCLA researchers achieved a limit-of-detection of 1 colony forming bacterium per 1 Liter of water sample under 9 hours of total test time, demonstrating a time saving of more than 12 hours for bacteria detection as compared to the gold-standard EPA methods. These results highlight the transformative potential of this AI-powered holographic imaging platform, which not only enables highly sensitive, rapid and cost-effective detection of live bacteria, but also provides a powerful and versatile tool for microbiology research.