Artificial neural networks now able to help reveal a brain’s structure
Digital image analysis steps up to the task of reliably reconstructing individual nerve cells
The function of the brain is based on the connections between nerve cells. In order to map these connections and to create the connectome, the “wiring diagram” of a brain, neurobiologists capture images of the brain with the help of three-dimensional electron microscopy. Up until now, however, the mapping of larger areas has been hampered by the fact that, even with considerable support from computers, the analysis of these images by humans would take decades. This has now changed. Scientists from Google AI and the Max Planck Institute of neurobiology describe a method based on artificial neural networks that is able to reconstruct entire nerve cells with all their elements and connections almost error-free from image stacks. This milestone in the field of automatic data analysis could bring us much closer to mapping and in the long term also understanding brains in their entirety.
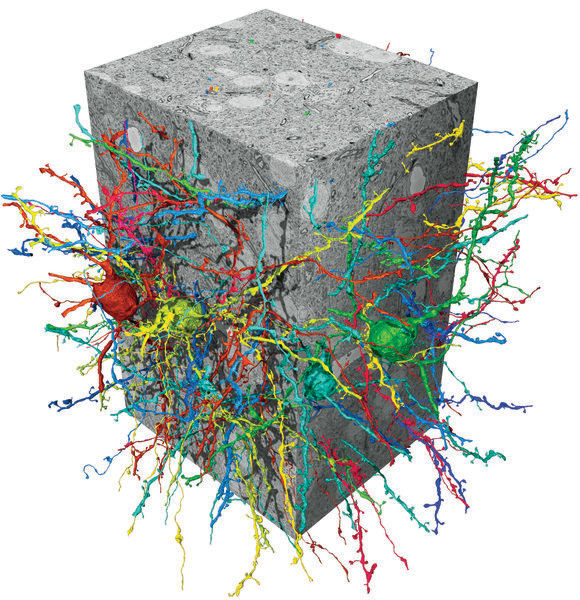
Flood-filling network (FFN) neuron reconstructions in a songbird basal ganglia SBEM data set.
MPI für Neurobiologie/ Kornfeld
Compared to the brain, artificial neural networks use vastly simplified “nerve cells”. Artificial intelligence based on these networks has nevertheless already found countless applications: from self-driving cars to quality control to the diagnosis of disease. However, until now the algorithms were too imprecise for very complex tasks such as the mapping of individual nerve cells with all their ramifications and contact points from a three-dimensional image of a brain.
“The cell structures that the computer generated from our electron microscopy images simply had too many mistakes”, relates Jörgen Kornfeld from the Max Planck Institute of Neurobiology in Martinsried. “In order to be able to work with these data, everything first had to be ‘proofread’.” That would require a lot of work: 11 whole years for an image stack with just 0.1-millimeter edge lengths. “That’s why we needed to find something better”, says Kornfeld. The best tools — at least currently – are the flood-filling networks (FFNs) developed by Michal Januszewski and colleagues at Google AI. A dataset from the brain of a songbird recorded already years earlier by Kornfeld and which he had partially analyzed by hand, played a big role in this development. The few cells that are carefully analyzed by humans represent the ground truth with which the FFNs first learned to recognize what a true structural protrusion looks like. On the basis of what has been learned, the remainder of the dataset can then be mapped with lightning speed.
Collaboration between computer scientists and biologists is nothing new in the department led by Winfried Denk. The leader of the Google research group, Viren Jain, was a PhD student at MIT in 2005 when Denk turned to Jain’s supervisor, Sebastian Seung. Denk wanted Seung’s help with the analysis of datasets generated using a method that had just been developed in Denk’s department. At that time in the department, Kornfeld was engaged in writing a computer program for data visualization and annotation. Kornfeld, whose research increasingly combines neurobiology and data science, was mainly responsible for developing the “SyConn” system for automatic synapse analysis. This system – like the FFNs – will be indispensable for extracting biological insights from the songbird dataset. Denk regards the development of the FFNs as a symbolic turning point in connectomics. The speed of data analysis no longer lags behind that of imaging by electron microscopy.
FFNs are a type of convolutional neural network, a special class of machine learning algorithms. However, FFNs also possess an internal feedback pathway that allows them to build on top of elements that they have already recognized in an image. This makes it significantly easier for the FFN to differentiate internal and external cellular structures on adjacent image elements. During the learning phase the FFN learns not only which staining patterns denote a cell border, but also what form these borders typically have. The expected savings made in terms of time needed for human proofreading through the use of the FFNs certainly justifies, according to Kornfeld, the greater computing power needed in comparison to currently used methods.
It now no longer seems completely inconceivable to record and analyze extremely large datasets, up to an entire mouse or bird brain. “The upscaling will certainly be technically challenging,” says Jörgen Kornfeld, “but in principle we have now demonstrated on a small scale that everything needed for the analysis is available.”